Object Detection
→ classification 뿐만 아니라 발견된 위치까지 찾아줌
Outline
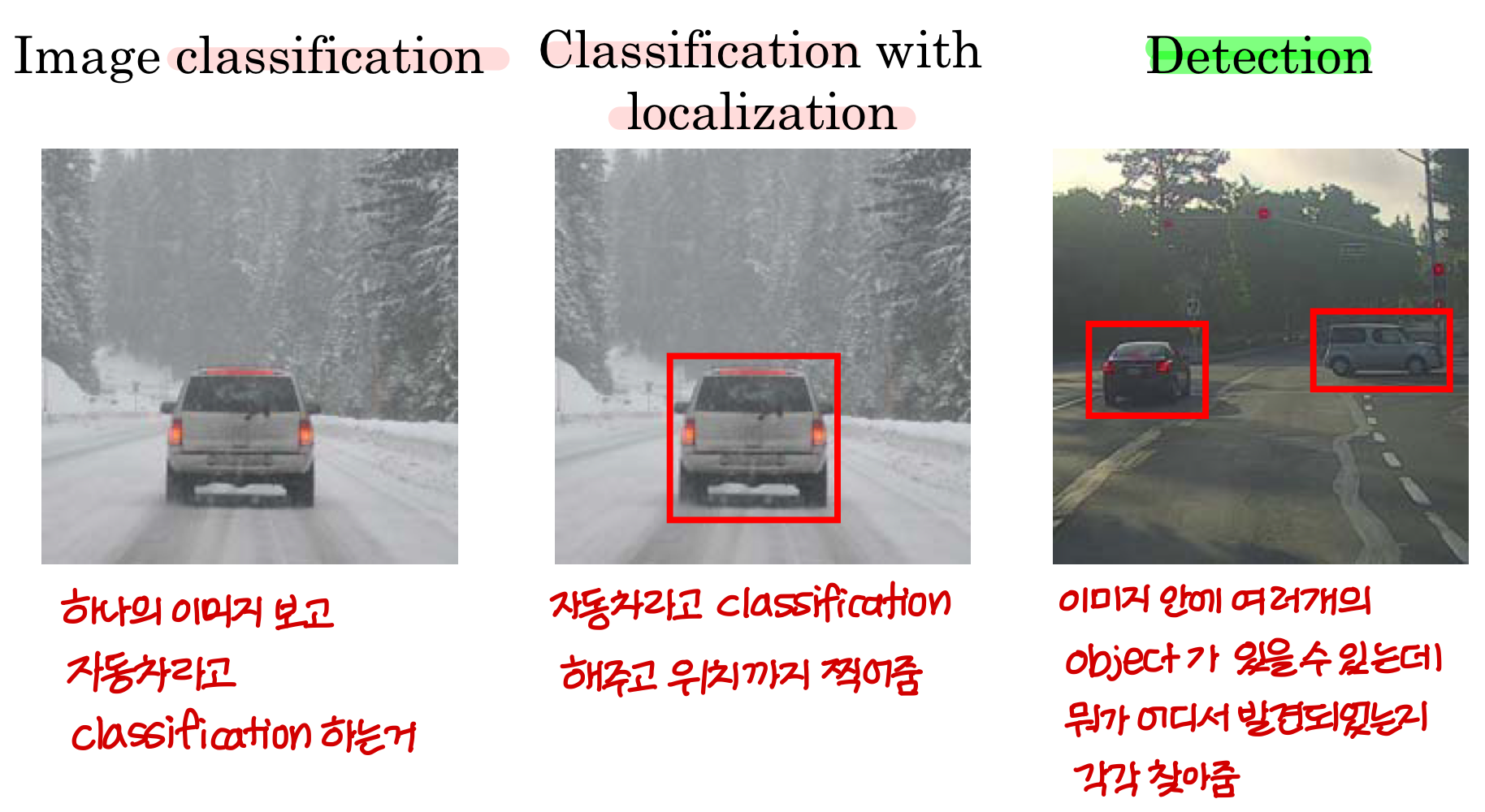
Classification with localization
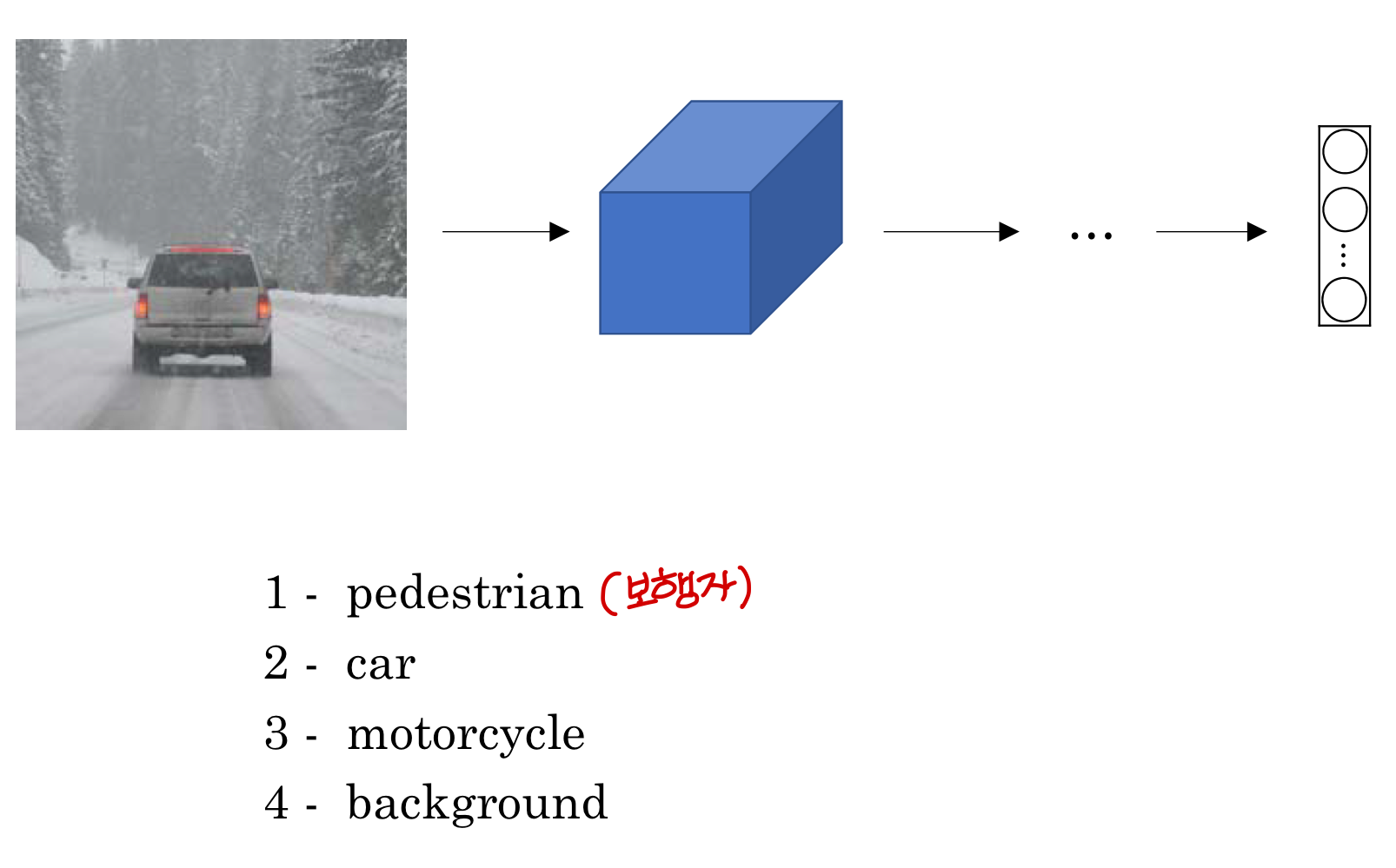
→ 클래스 이렇게 4개 (보행자, 차, 오토바이, 배경)
Car detection example
Turning FC layer into convolutional layer(FC layer를 convolutional layer로 전환)
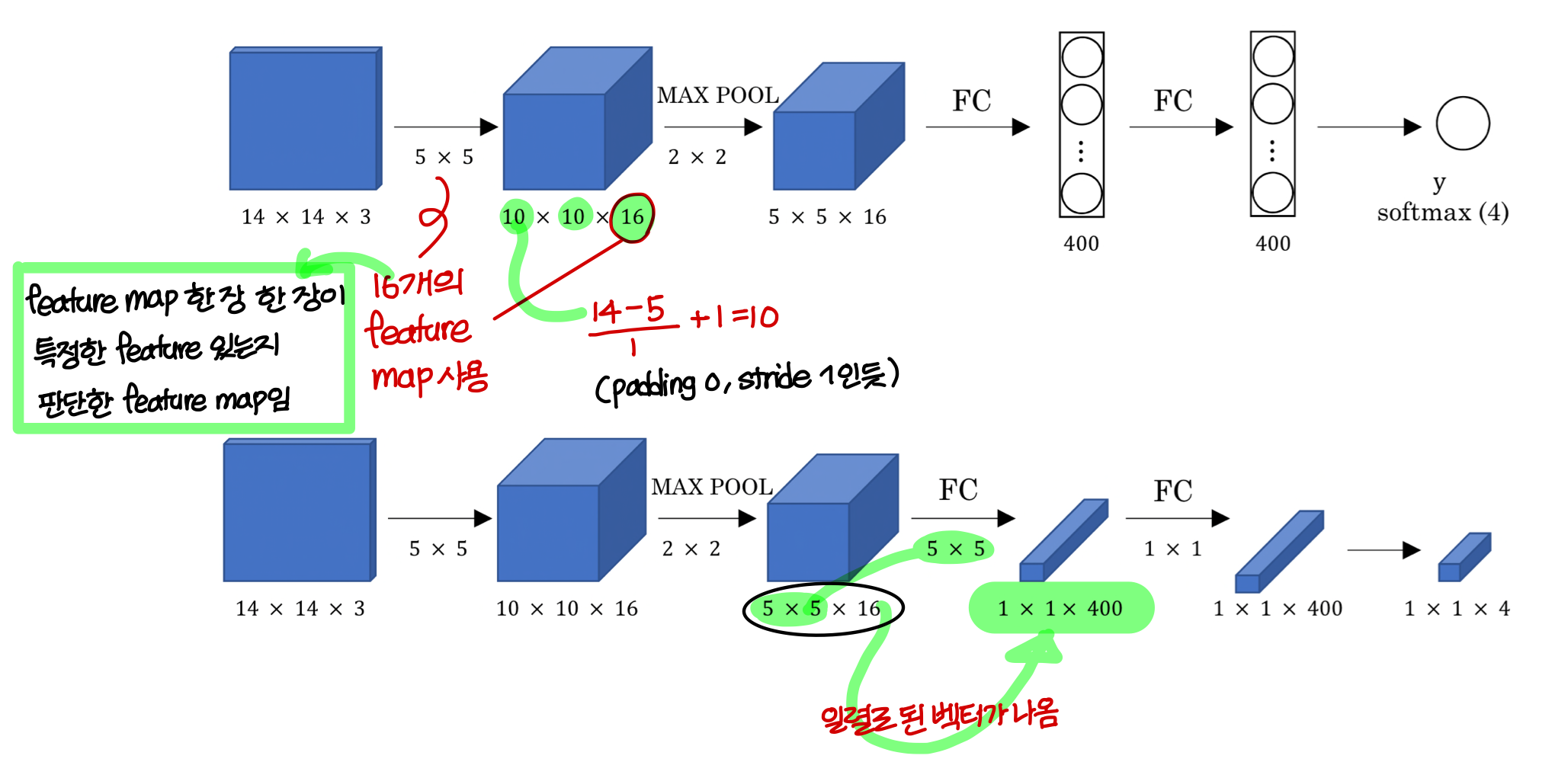
Convolutional implementation of sliding window
- sliding window는 filter의 stride와 다름
→ sliding window 하나하나에 대해서 stride 만큼 움직이면서 filter 적용되겠지
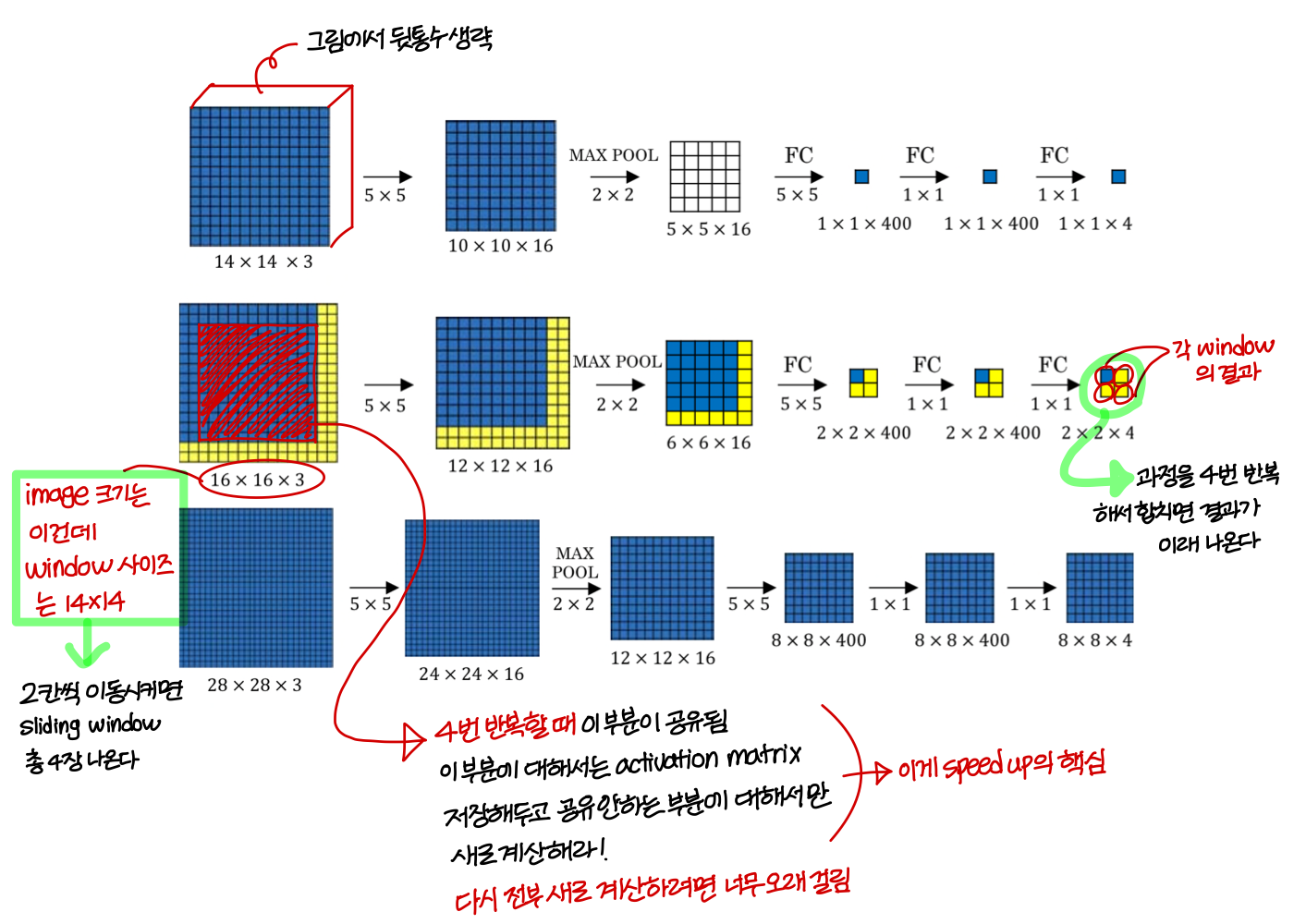
- sliding window를 작게 할수록 좋은 것도 아님
- sliding window 또 너무 크게 하면 그냥 CNN이랑 똑같음
- 그리고 object가 sliding window 상의 어디에 위치하는지 알기 위해 localization regression 필요
Output accurate bounding boxes
YOLO algorithm
defining the target label y
- 의 합은 1이지만 전체의 합은 1이 아님
→ 그래서 전체에 대해 sum of square funcition을 사용하거나
(sum of square: 이건 임의의 함수 fitting 시킬 때 사용하는 loss 함수)
→ 아니면 sum of square + cross-entropy
제외한 부분에 대해서는 sum of square
에 대해서는 cross entropy
- 의 의미는? object가 있을 확률
→ 정확히 말하면 물체의 bounding box의 무게중심이 grid 안에 존재할 확률!
→ 학습시킬 땐 bouding box의 무게중심이 grid 안에 존재하면 1, 아니면 0
YOLO algorithm
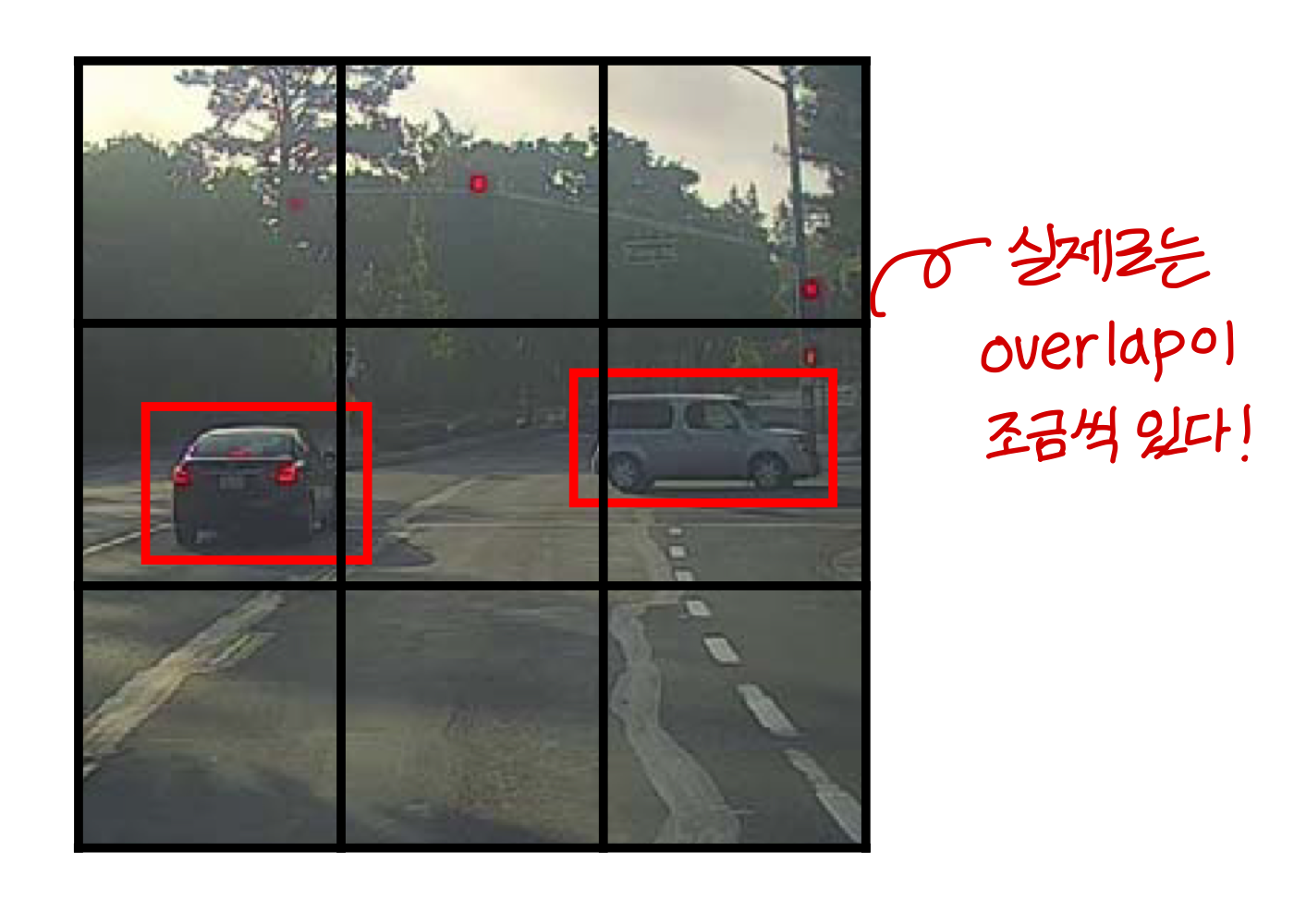
Evaluating object localization
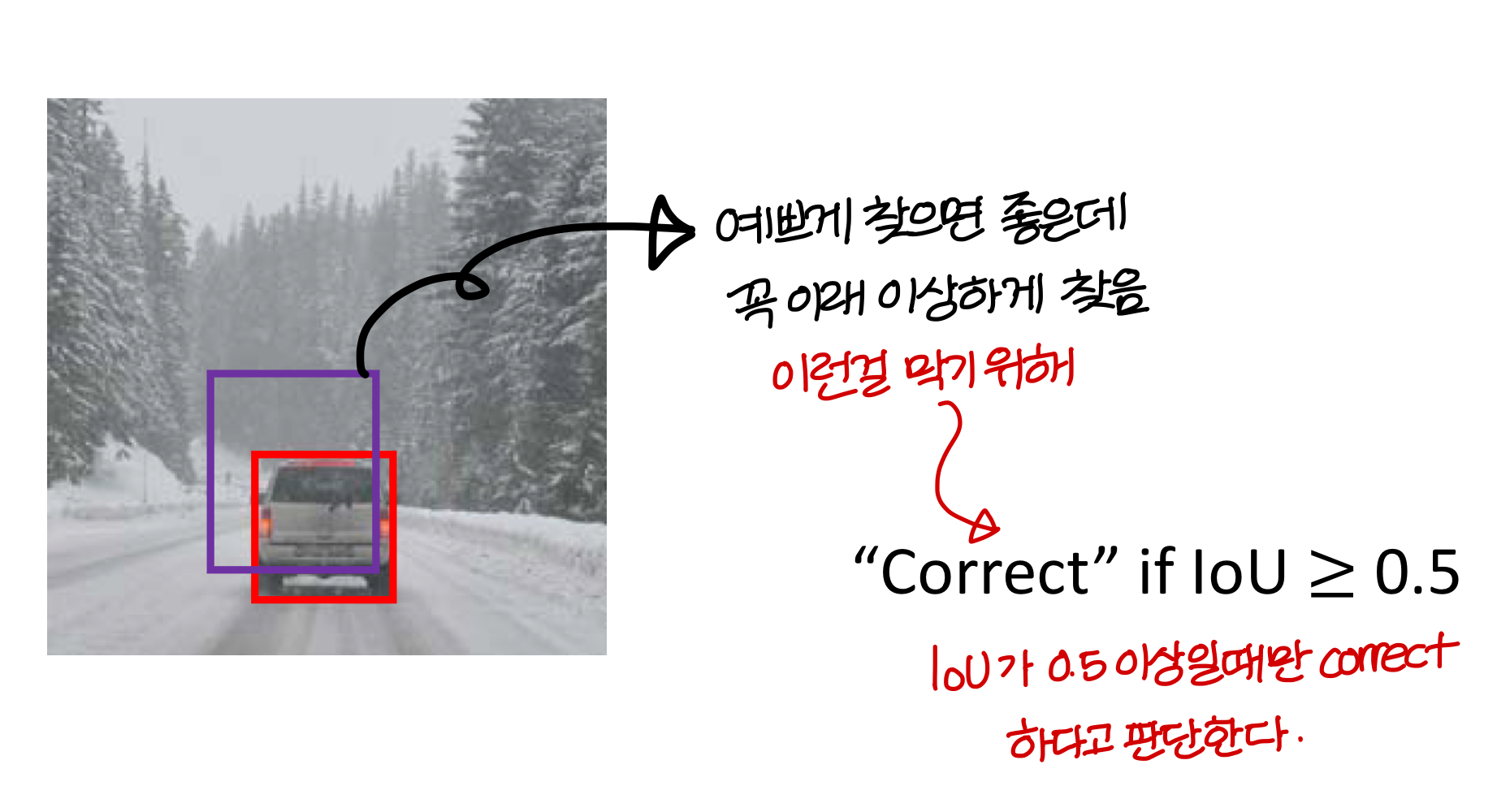
Non-max suppression example
- overview
→ 위의 방식으로 bounding box마다 점수를 매긴다!
→ 5개나 찾겼는데 여기서 제일 점수 높은 애를 고르고, 걔랑 일정 수준 이상으로 overlap 된 애들 제거함
그리고 남은거 중에 또 점수 제일 높은 애를 고르고, 걔랑 일정 수준 이상으로 overlap 된 애들을 제거한다
Overlapping objects
- Anchor box 2개 사용하게 되면
→ y가 이렇게 정의된다
→ 학습시킬 때부터 y를 이렇게 둬야한다!
→ 위의 사진의 상황인 경우에는 training data의 y가 이렇게 세팅됨
Training
- object 아무것도 없을 땐(Two anchor box 사용하는 경우)💡don’t care 값 다 0으로 놓고 loss function 적용하면 어떻게 되나?
→ 굳이 이거 fitting 시킨다고 다른거 희생할 수 있음
→ 0으로 놓고 하는것보다 그냥 don’t care로 놓고 계산 안하는 것이 결과에 좋음
Outputting the non-max suppressed outputs
anchor box와 non-max supression을 썼을 때 결과가 예쁘게 만들어진다!
→ anchor box 2개 썼으므로 각 grid마다다
bounding box 2개씩 예측한다
→ non-max supresssion에서 배운대로
일단, low probability prediction인 애들을 제거함
→ 그리고 non-max suppression 방식으로
final predictions을 만들어낸다
Region proposal: R-CNN
- sliding window 방식으로 하는건 사람이 하는거랑 좀 괴리가 있음
→ 사람이 object를 인식할 때 한 영역씩 쪼개서 보진 않으니까
→ 사람은 object를 인식할 때 일단 크게 보고, 있을 만한 부분을 자세히 봄
- 그래서 일단 segmentation을 함
→ segmentation 기법을 사용해 픽셀별로 classfication을 한다!
→ 이렇게 segmentation 된 거 오려서 각 영역에 대해서 CNN을 적용함
Uploaded by N2T