728x90
728x90
하고자 하는 것
- Audio Input과 Emotion labels을 받으면, 해당 emotion에 적절하면서도 audio input에 맞는 speech facial animation을 생성할 수 있는 EMOTE framework 제안
Dataset
- emotional speech에 대한 dataset은 존재하지 않음. 그래서 emotional video dataset인 MEAD dataset에서 reconstruction method 사용해서 생성한 data 사용.
- MEAD dataset에 포함된 감정 label을 사용하는 것이 아니라 emotion feature를 따로 extract해서 사용
- EMOCA’s public available emotion recognition network를 사용해서 emotion feature extract
- emotion feature는 (emotion class, valence, arousal)로 구성
- emotion class: 9개의 감정 class 중 하나로 분류 (natural, happiness, sadness, surprise, fear, disgust, anger, and contempt)
- valence: 감정이 얼마나 긍정적인지 또는 부정적인지를 나타내는 값
- arousal: 감정의 강도나 흥분 수준을 나타내는 값
- EMOCA의 network를 다 학습시킨 후, prediction head를 버리고 final ResNet layer의 output을 feature vector로 사용
- 영상에 대한 emotion feature를 구하기 위해 sequence of emotion features를 입력으로 받아 video 전체의 emotion classification vector(8차원)와 video emotion feature(256차원)를 출력하는 network 따로 학습시킴. 이렇게 생성한 emotion 정보를 가지고 dataset 구성
- emotion feature는 (emotion class, valence, arousal)로 구성
Method
두 단계의 학습을 거침
- 1️⃣ FLINT 학습: temporal variational autoencoder를 학습시키고, latent space를 motion prior로 사용
- 2️⃣ EMOTE 학습: mapping 시킬 때 주어진 target emotion과 intensity(mild, medium, or high)에 conditioned되도록 mapping시킴. speech audio와 motion prior의 latent space를 mapping시키는 regressor를 학습시킴.
1️⃣ Facial Motion Prior: FLINT
- Input: T frame의 FLAME parameter sequence (53, T)
- Architecture
- VAE framework를 확장해서 transformer encoder를 사용
- Facial motion이 frame간에 independent 하지 않음. 그래서 lower-dimensional latent space에서 표현(d, T/q)
- Loss: KL divergence loss와 Reconstruction loss 사용
- KL divergence loss: latent sequence(d, T/q)를 한번에 다루는게 아니라 sequence의 element 각각 latent와 sigma를 사용해 loss값을 계산하였음
- Reconstruction loss: mesh 간의 vertex coordinates 차이를 가지고 계산한 MSE 값을 reconstruction loss로 사용
2️⃣ Emotional Speech-Driven Animation: EMOTE
- 동작설명
- encoder에서는 Wav2Vec 2.0 사용 (raw audio input에서 audio feature 뽑아내는 network)
- Wav2vec 2.0을 통과한 audio feature sequence와 style vector를 concatenate 시킴
S는 styling function(input condition c를 linear projection 시킨거)
c는 input condition으로 training할 때는 ground-truth emotion type, emotion intensity, speaker ID로 세팅됨
- style feature와 합쳐진 speech feature는 motion prior의 latent space로 mapping됨
- 결과적으로 latent sequence z^{1:T/q}를 얻게 됨
- 이 latent seqeunce가 미리 학습시킨 decoder에 제공되고 decoder는 output으로 FLAME parameter를 출력
- training 할 때, ground truth와 predicted geometry를 미분가능한 renderer를 사용해 각각 렌더링
- Estimated face mesh sequence를 lip reading network와 video emotion network로 넘겨줌.
- 즉 결과적으로 network는 vertex sequence, lip-reading feature sequence, video emotion feature를 출력
- Training 설명
1. Reconstruction loss
FLINT의 학습에서와 동일하게 mesh 상의 vertex coordinate 차이로 구함
2. Video emotion loss
original video를 video emotion network를 통과하도록 하여 나온 emotion feature와 EMOTE의 결과로 출력되는 emotion feature의 distance만큼 penaliz
3. Lip-reading loss
Video emotion loss와 마찬가지로 원본 데이터를 렌더링한 이미지와 network가 출력하는 flame 값을 사용해 렌더링한 이미지를 각각 Lip reading network에 통과시켜 나온 lip-reading feature의 distance만큼 penalize
4. Disentangling emotion and content
그냥 단순히 conditioning 하는걸로 emotion-content가 잘 분리되어 학습되지 않음
그래서 새로운 Emotion-content disentanglement mechanism 사용
training 중에 다른 emotion을 가지는 2개의 sequence를 골라 그들의 emotion condition을 서로 바꾸어버서 입력으로 넣어줌 (speech audio는 그대로)
이러한 세팅에서 lip-reading loss(L^dis_lip)는 원본 영상을 사용해서 계산하고, video-emotion loss(L^dis_emo)는 바꾼 대상의 원본 영상을 사용해서 계산함
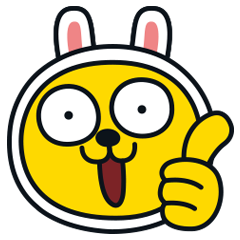
728x90
728x90